Our research
Cancers are heterogeneous in terms of mutation profile, cell type of origin, and micro-environment, and finding effective treatments for each individual cancer is the aim of precision cancer medicine. This is challenging since cellular processes are highly interconnected and complex. We develop deep learning models of the molecular networks of cells, to identify systems-level mechanisms in cancer.
Deep learning models excel at finding complex relations in large quantities of data, but are not always easy to interpret. To address this, we constrain the models, so that their predictions align with physical interactions between biomolecules. The models cover metabolism, signal-transduction and gene regulation and are trained on high throughput datasets such as transcriptomics, metabolomics, and proteomics from different cell types, interventions, and conditions.
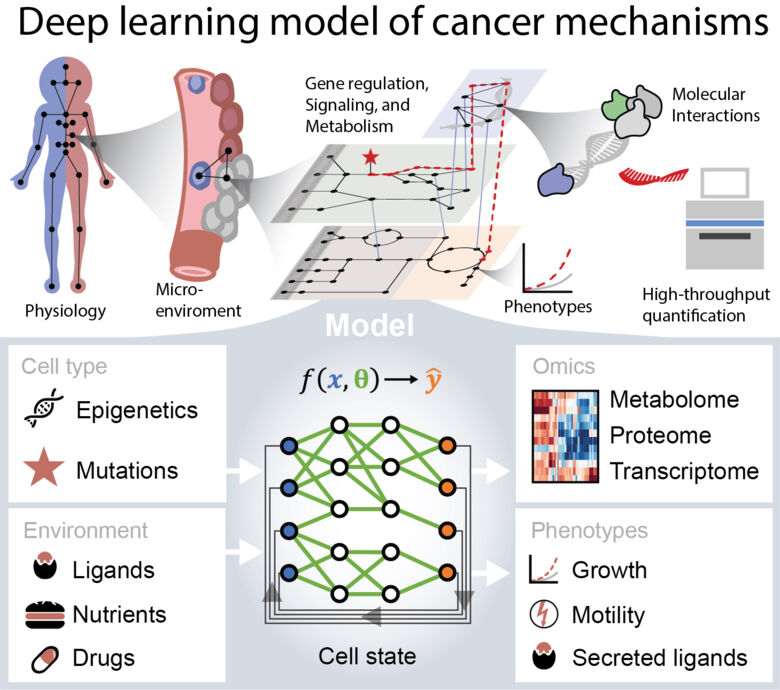
Using our models, we aim to find new drug targets and biomarkers, predict resistance mechanisms, and improve the understanding cell-cell interactions in the tumor microenvironment. We are particularly interested in treating cancer-stroma interactions in pancreatic cancer. Our long-term goal is to enable computer aided design of cancer medicine.