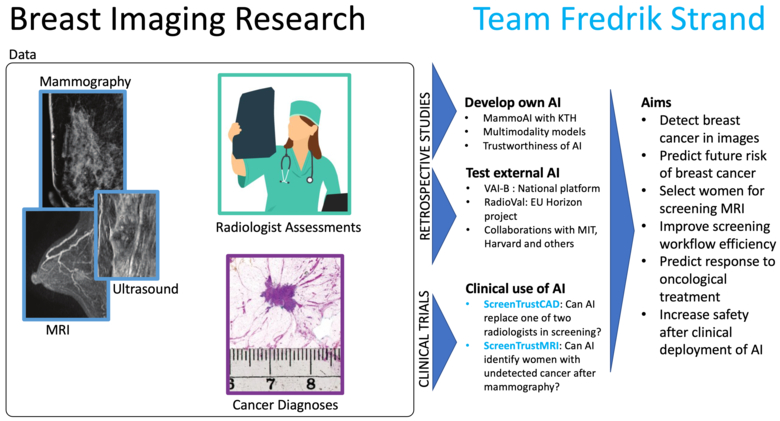
Introduction
We are living in exciting times. The use of Artificial Intelligence is on the rise. The true limits to what can be achieved are not yet known. Our focus are the more than 600,000 women who die of breast cancer each year in the world.
Image-based AI for precision screening
Early detection is the most important measure, until now achieved by population-wide mammography screening every 1 to 3 years for women in a certain age groups. Nevertheless, around 30 percent of the cancers diagnosed for women who participate in screening are clinically detected in the interval between two screenings; and nearly 15 percent of screen-detected cancers are 2 cm or larger. Employing AI to tailor and improve the screening could bring the benefit of early detection to more women keeping in mind the scarcity of radiological resources - image-based AI for precision screening.
Image-based AI for precision medicine
Oncological treatment options have become more diverse and have contributed to prolonged survival. However, each cancer resides in its own microenvironment of evolutionary pressure, which means no two cancers are alike. The use of preoperative, neoadjuvant, medical treatment has increased since it offers an opportunity to study how the cancer reacts to a certain medication while it remains in the breast. The need to predict which treatment is likely best for each patient, and the need to study how the cancer reacts over time, puts increasing demands on the precision of imaging and on cross-disciplinary analysis between radiology (non-invasive imaging of the whole breast, and the whole cancer) and pathology (invasive sample of the cancer). In addition, surgery has become more precise and less extensive. Employing AI on medical images could improve mapping of the tumor extent, predicting the best therapy, and continuously assessing the response - image-based AI for precision treatment.
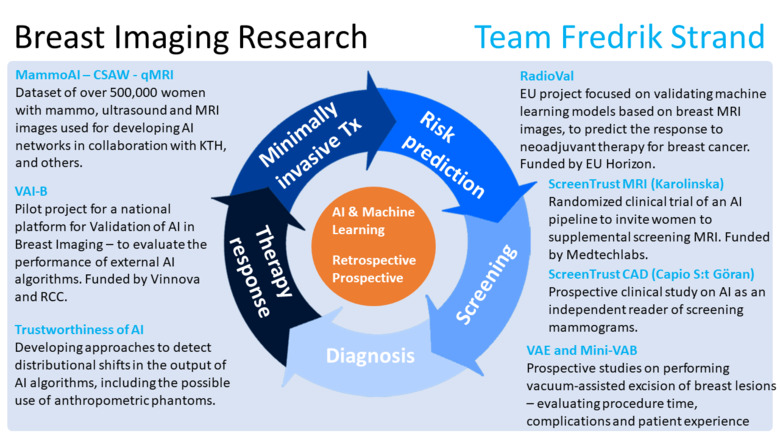
Research focus
Our studies are cross-disciplinary. Since 2016 we have built a strong collaboration with computer science researchers at the Royal School of Engineering (KTH) in Stockholm.
Cancer detection - ScreenTrust CAD
To explore what happens when AI is employed in clinical practice, we are heading the clinical study ScreenTrust CAD, with clinical partner Capio S:t Göran Hospital, in which we explore the use of AI as an independent reader of screening mammograms. The study is a paired screen-positive design to determine whether AI plus one radiologist is non-inferior to two radiologists in double-reading. In addition, we collaborate with Jennifer Viberg Johansson at the Center for Research ethics and Bioethics at Uppsala University to understand the qualitative aspects of AI interaction among radiologists and among screening participants.
Undetected cancer - ScreenTrust MRI
We have jointly developed deep networks that can be used to assess for each woman: the risk of getting breast cancer in the future, and how difficult it would be to visualize an early cancer in the mammogram. Those algorithms are now explored in our ongoing randomized clinical study ScreenTrustMRI which is mainly financed by Medtechlabs. The primary end-point is to determine whether our approach reduces the number of interval cancers and advanced cancers compared to mammography-only. We will also determine how many cancers are detected per 1000 women screened by MRI to enable a cost effectiveness analysis compared to regular screening.
Algorithm validation - VAI-B
Not all AI algorithms are alike. In a JAMA Oncology study in 2020, we demonstrated major differences between three commercial algorithms in our retrospective dataset of mammograms and clinical outcomes. The size of our dataset is around 500,000 mammography exams and 3,500 MRI exams and more than 1 million screening assessments. Continuing our independent evaluation work, we are heading the national project VAI-B aiming to establish a Swedish platform for validation of AI algorithms in breast imaging. The project is a collaboration between the regions and universities in Stockholm, Malmö and Linköping; and the breast cancer association. The project is funded by Vinnova and the Regional Cancer Centers. The aim is to create a scalable platform delivering value to hospitals: impact assessment and evaluations, as well as to AI developers: external validation and benchmarking.
Treatment response prediction - RadioVal
Recently, the EU project RadioVal was awarded funding in the Horizon Europe program. We form part of the consortium, in which we will be responsible for clinical validation of machine learning algorithms for the prediction of therapy response for neoadjuvant treatment. This work is carried out in collaboration with breast oncologist Theodoros Foukakis.
Multi-modality algorithms - qMRI
Our work has to a large extent been based on mammograms, but now we are adding other modalities such as MRI. Together with KTH we will start developing multi-modality networks possibly also including pathology data and images. The aim is to annotate more than 3000 MRI Breast exams, and to train networks to detect cancer, predict risk, and to predict histopathological characteristics, as well as to predict response to neoadjuvant therapy.
AI trustworthiness
An important aspect of AI algorithms is what enables humans to trust them – or not. Previously it was popular to demand that algorithms should be able to explain how they reached a certain conclusion. Now, the focus has shifted towards trustworthiness. This could mean that even if the AI algorithm is not explainable in human terms, it can be demonstrated that it works to an extent that humans require, e.g., that the algorithm is accurate, robust, transparent, et cetera. It could also mean continuous surveillance of AI algorithms in clinical use to detect when they diverge from the expected performance. We are involved, in collaboration with KTH, to examine this important topic.
Our main funders are currently Medtechlabs, Regional Cancer Centers in Collaboration, Vinnova, Region Stockholm, EU Horizon Europe, and the Swedish Breast Cancer Association.