Biostatistics Team
The Biostatistics Team aims to gather statistical and methodological expertise across the Department of Global Public Health and Centre for Epidemiology and Community Medicine.
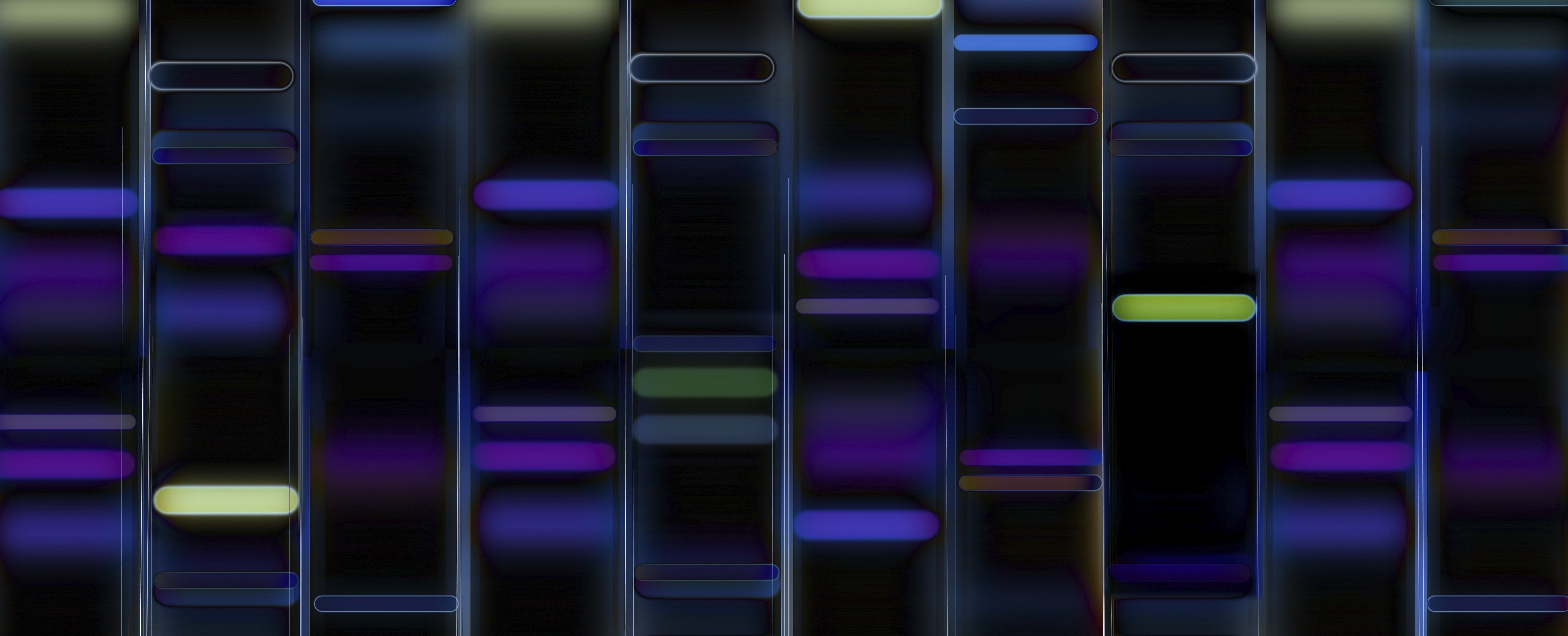
Our goal is strengthening our research capacity in epidemiology and public health and exploiting the richness and interdisciplinary work carried out within the department.
The objectives of the team are to:
- interact with the research groups
- provide strategic quantitative methodological support
- teach biostatistics in undergraduate and postgraduate courses
- organize regular workshops and short courses on special topics
- develop sound and innovative statistical methods
- share user-friendly statistical tools
- offer statistical support for grant proposals
- co-supervise doctoral students
Group members
Nicola Orsini
Head of Biostatistics TeamRobert Thiesmeier
Phd Student;Affiliated to ResearchAffiliated
- Hugo Sjöqvist, Hugo.sjoqvist@ki.se
- Filip Andersson, filip.andersson@ki.se
- Michael Lundberg, michael.lundberg@ki.se
- Per Tynelius, per.tynelius@ki.se
- Susanne Wicks, susanne.wicks@ki.se
- Henrik Dal, henrik.dal@ki.se
- Gunnar Branden, gunnar.branden@ki.se
- Zangin Zeebari, zangin.zeebari@ki.se
Projects
Dose-response meta-analysis
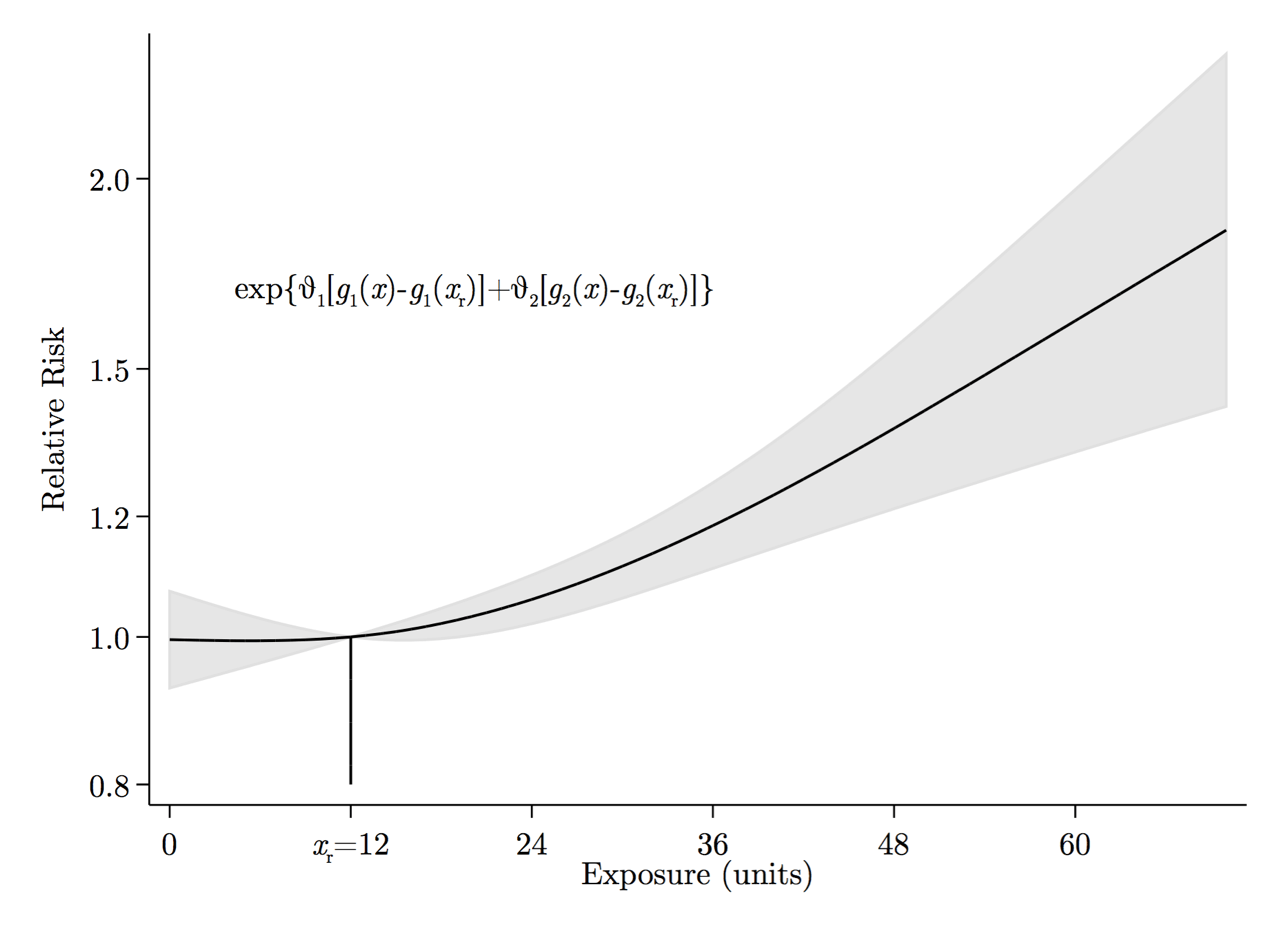
A dose-response analysis describes the changes of a response across levels of a quantitative factor. A meta-analysis of dose-response (exposure-disease) relations aims at identifying the summary trend emerging from multiple studies trying to answer the same research question. Examples of questions usually asked are:
- Is there any association at all?
- Is the response changing approximately at a constant rate throughout the observed exposure range?
- Is there any substantial change in the outcome beyond a certain exposure level?
We focus on addressing these questions by developing statistical methods for the analysis of multiple summarized data arising from experimental or observational data.
A percentile approach for time-to-event analysis
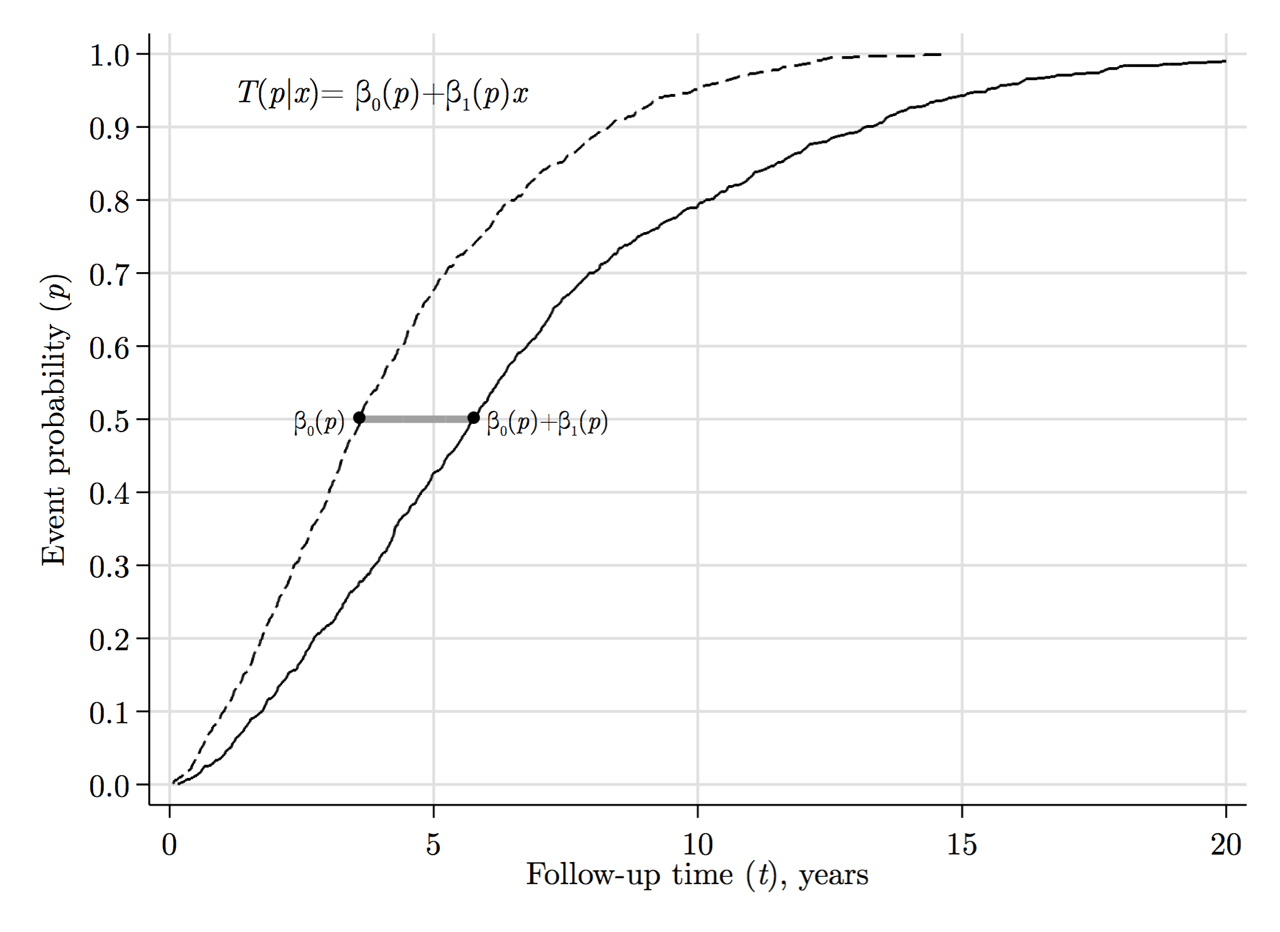
The percentile can be an attractive measure because it makes explicit the link between two pieces of information: survival probability and a specific time point. Results from prospective studies can be presented in terms of differences or ratios of survival times, facilitating both interpretation and communication of scientific findings.
The introduction of a statistical technique to estimate conditional survival percentiles has substantially enriched its potentialities and eased its application in research. We exploit the potential of the percentile in epidemiological and public health research.
Time-series analysis to assess the effectiveness of interventions
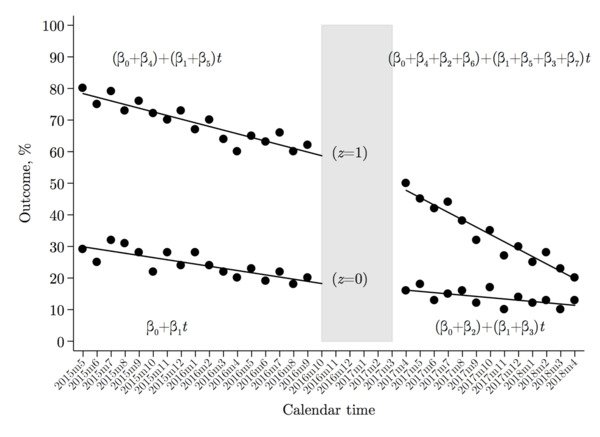
Randomized controlled trials are considered the ideal approach for assessing the effectiveness of interventions. Not all interventions, however, can be assessed with a trial simply because it is not affordable, ethical, or possible. In addition, even well designed randomized trial can be susceptible to biases, particularly when generalizing results to “real world” settings.
Interrupted time-series analysis is a suitable evaluation approach when a single unit (N=1) is being studied (i.e., individual, city, state, country), the outcome is serially ordered as a time series, and multiple observations are collected at equally space intervals.
We focus on methodological questions such as how to adjust for correlated outcome measures and regular seasonal fluctuations that could potentially bias the time-series analysis, how to deal with time-varying confounding due to changes in outcome coding, co-interventions, or changes in the population under study.